AI Agents and RAG
Inngest offers tools to support the development of AI-powered applications. Whether you're building AI agents, automating tasks, or orchestrating and managing AI workflows, Inngest provides features that accommodate various needs and requirements, such as concurrency, debouncing, or throttling (see "Related Concepts").
Quick Snippet
Below is an example of a RAG workflow (from the "AI in production" blog post), which uses Inngest steps to guarantee automatic retries on failure.
./inngest/functions.ts
export const userWorkflow = inngest.createFunction(
fnOptions,
fnListener,
async ({ event, step }) => {
const similar = await step.run("query-vectordb", async () => {
// Query a vectorDB for similar results given input
const embedding = createEmbedding(event.data.input)
return await index.query({ vector: embedding, topK: 3 }).matches
})
const response = await step.run("generate-llm-response", async () => {
// Inject our prompt given similar search results and event.data.input
const prompt = createAgentPrompt(similar, event.data.input)
return await llm.createCompletion({
model: "gpt-3.5-turbo",
prompt,
})
})
const entities = await step.run("extract-entities-hf", async () => {
// Extract entities from the generated response using Hugging Face's named entity recognition model
let pipe = await pipeline(
"entity-extraction",
"Xenova/bert-base-multilingual-uncased-sentiment"
)
return await pipe(response)
})
const summary = await step.run("generate-summary-anthropic", async () => {
// Generate a summary document using the extracted entities and the Anthropic API
const anthropic = new Anthropic()
const anthropicPrompt = `The following entities were mentioned in the response: ${entities.join(
", "
)}. Please generate a summary document based on these entities and the original response:\n\nResponse: ${response}`
return await anthropic.messages.create({
model: "claude-3-opus-20240229",
max_tokens: 1024,
messages: [{ role: "user", content: anthropicPrompt }],
})
})
await step.run("save-to-db", async () => {
// Save the generated response, extracted entities, and summary to the database
await db.summaries.create({
requestID: event.data.requestID,
response,
entities,
summary,
})
})
}
)
App examples
Here are apps that use Inngest to power AI workflows:
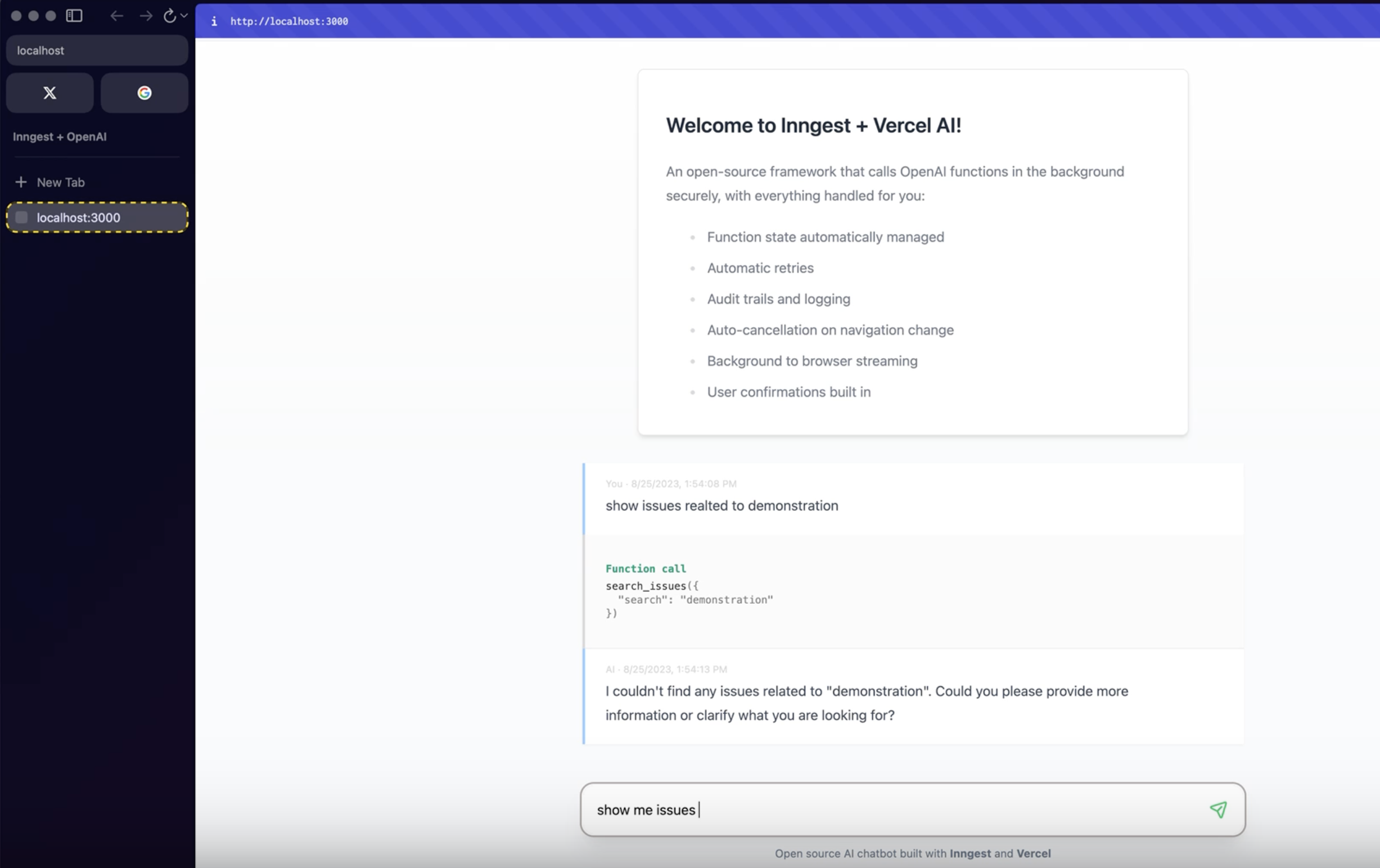
Integrate AI agents with Inngest
AI-powered task automation in Next.js using OpenAI and Inngest. Enhance productivity with automated workflows.
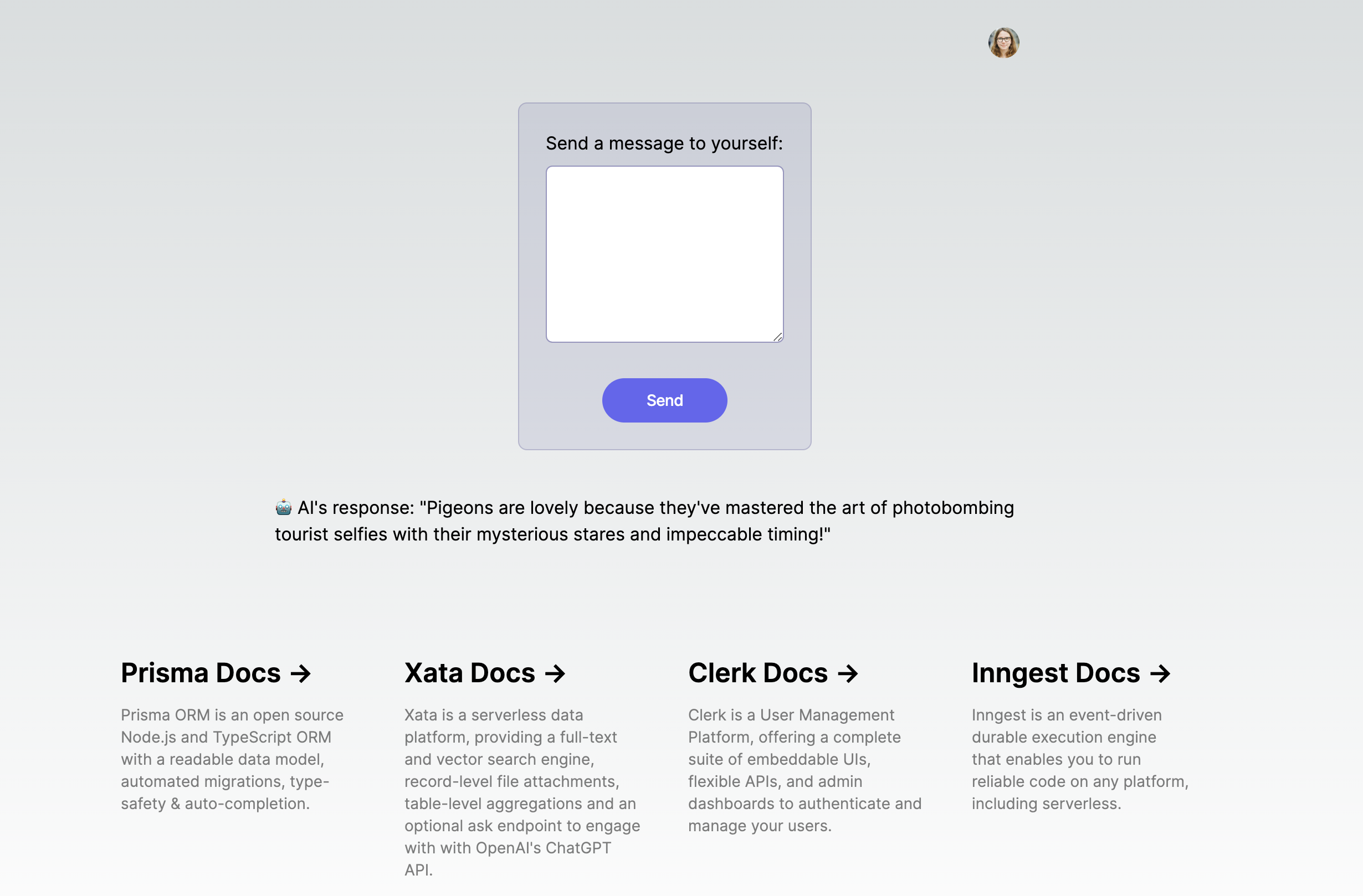
PCXI starter
A boilerplate project for the PCXI stack featuring an OpenAI call
Resources
Check the resources below to learn more about working with AI using Inngest:
Related concepts
- Concurrency: control the number of steps executing code at any one time.
- Debouncing: delay function execution until a series of events are no longer received.
- Prioritization: dynamically execute some runs ahead or behind others based on any data.
- Rate limiting: limit on how many function runs can start within a time period.
- Steps: individual tasks within a function that can be executed independently with a guaranteed retrial.
- Throttling: specify how many function runs can start within a time period.